From Chatbots to Human-Like Interactions: The Ultimate Guide to Conversational AI Success
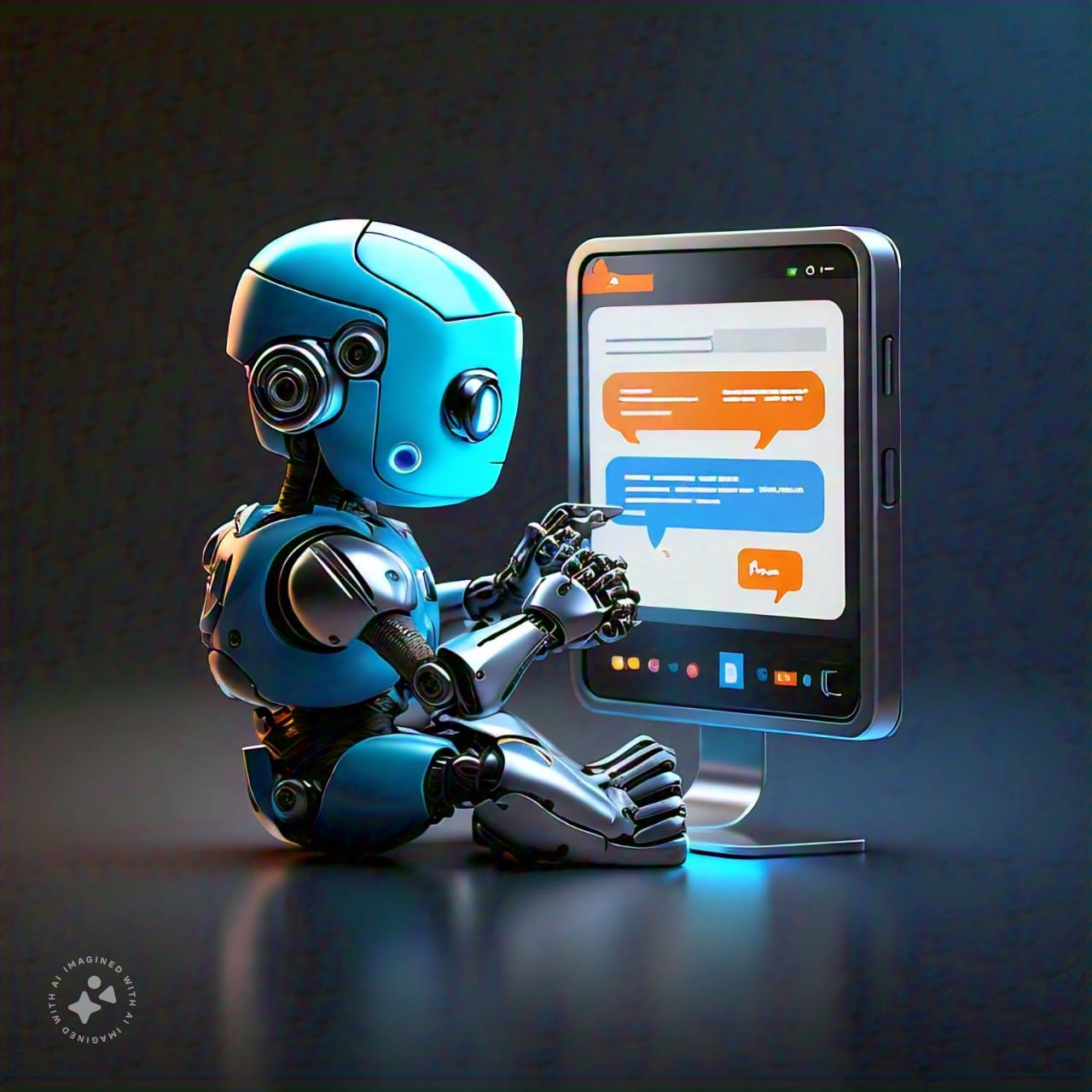
The global conversational AI market size is projected to reach $13.9 billion by 2025, growing at a Compound Annual Growth Rate (CAGR) of 22.7% from 2020 to 2025 (Source: MarketsandMarkets). This growth is propelled by the increasing use of Generative AI and machine learning to streamline customer support, improve customer experience, and enhance operational efficiency. With significant advancements in natural language processing (NLP) and automation, conversational AI is reshaping communication in various industries, making it essential for organizations seeking to remain competitive and offer better services to it's customers.
As conversational AI becomes increasingly prevalent, customers' expectations for intuitive and personalized interactions continue to rise. To create AI applications that truly resonate with users, it's crucial to understand the key attributes that drive effectiveness. This blog examines the essential characteristics based on research [ Persona based conversational AI, Context retrieval conversational agent ] of conversational AI from a customer-centric viewpoint.
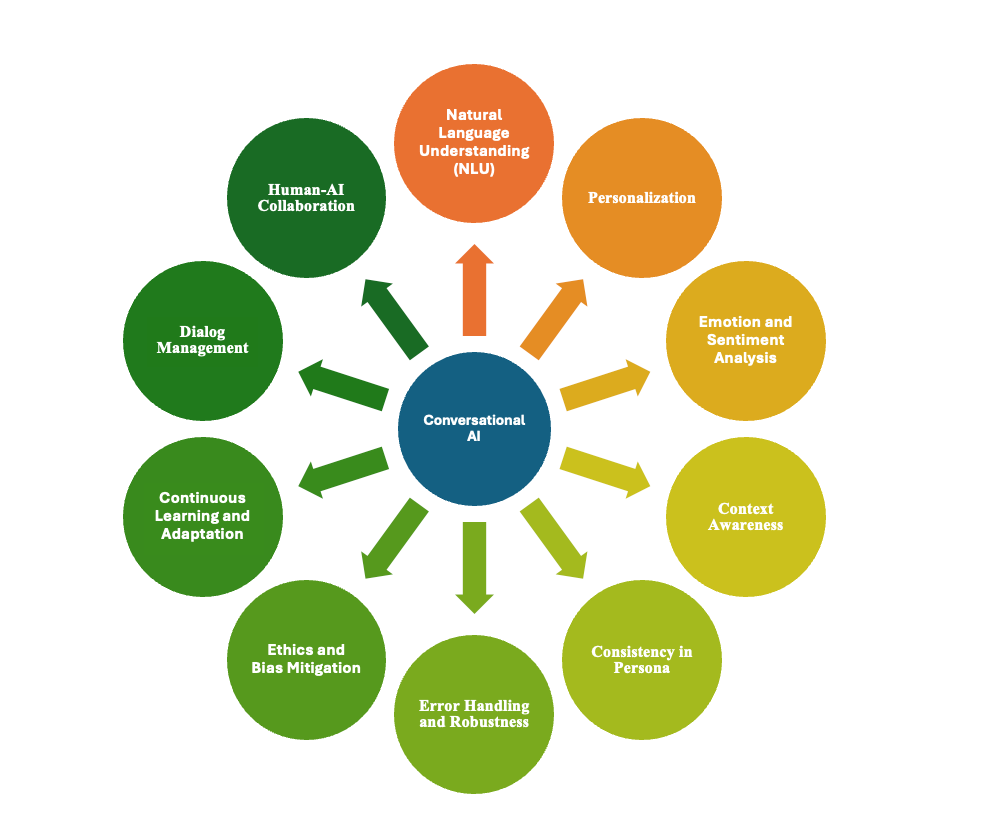
1. Context Awareness
Conversational AI systems need to comprehend the context in which the conversation occurs, including not only the content but also the context of previous interactions, external knowledge bases, and the environment of the user. This allows for more natural and accurate responses. Research points out the importance of context modeling for more coherent and continuous conversations.
Example: A customer contacts a bank's AI-powered chatbot to inquire about their account balance. The chatbot recalls their previous conversation, retrieves the relevant account information, and provides the balance without asking for redundant details.
Benefits:
- Streamlines conversations
- Reduces user frustration
- Enhances personalization
2. Natural Language Understanding (NLU)
A core component of any Conversational AI is its ability to understand natural language input. Advanced natural language understanding models like transformers are used to decipher user input with high accuracy. NLU helps in parsing queries, understanding intent, and even detecting sentiment or emotional tone in the conversation.
Example: A user asks a virtual assistant, "What's the weather looking like for my weekend getaway to NYC?" The AI understands the query, recognizes "weekend" as a reference to Saturday and Sunday, and provides the relevant weather forecast.
Benefits:
- Improves accuracy
- Enhances user experience
- Supports diverse languages and dialect
3. Personalization
Persona-based designs are enhanced when combined with personalization features that adapt the conversation to the user's unique preferences, past interactions, and personality traits. Studies show that this can significantly improve user engagement and satisfaction..
Example: An e-commerce AI chatbot suggests products based on a customer's purchase history and browsing behavior. "Hi John, we've got new arrivals in your favorite shoe brand. Would you like to explore?"
Benefits:
- Increases engagement
- Boosts conversions
- Fosters loyalty
4. Emotion and Sentiment Analysis
Understanding the emotional tone of a conversation allows Conversational AI to adjust its responses accordingly. Sentiment analysis can be used to detect whether the user is happy, frustrated, or neutral, which can significantly impact the quality of interaction.
Example: A customer complains to an AI-powered support agent about a delayed delivery. The AI detects frustration and responds with a calming message, "I apologize for the inconvenience. I'm here to help resolve the issue as quickly as possible."
Benefits:
- Enhances user satisfaction
- Reduces escalation
- Improves brand reputation
5. Consistency in Persona
Ensuring consistency in responses is critical, especially when the AI is designed to maintain a specific persona or brand voice. Research highlights that many LLM-based systems, such as some versions of GPT, still face challenges in maintaining persona consistency throughout interactions.
Example: A well-known tech brand's AI assistant maintains a friendly, approachable tone across all interactions, mirroring the brand's marketing and customer support.
Benefits:
- Reinforces brand identity
- Builds trust
- Enhances user experience
6. Error Handling and Robustness
Conversational AI must be able to gracefully handle misunderstandings, ambiguous queries, or out-of-scope requests by implementing fallback mechanisms and error-recovery strategies.
Example: A user asks a voice assistant to play a song with incorrect song title. The AI responds, "I didn't quite catch that. Can you please provide more details or try rephrasing the song title?"
Benefits:
- Minimizes frustration
- Ensures seamless interactions
- Maintains user engagement
7. Ethics and Bias Mitigation
Handling sensitive information requires robust ethical guidelines, including ensuring user privacy, secure data handling, and adhering to data protection laws. Ethical AI ensures fairness and minimizes biases in responses, particularly important for AI systems used in sensitive sectors like healthcare.
Example: A language model AI is designed to avoid generating responses that promote hate speech, stereotypes, or harmful content.
Benefits:
- Protects users and brand reputation
- Ensures compliance with regulations
- Fosters inclusive interactions
8. Continuous Learning and Adaptation
The system should learn and evolve over time, improving responses as more data is accumulated. Techniques like reinforcement learning, fine-tuning on user data, and active learning help conversational systems improve gradually.
Example: An AI-powered customer support platform analyzes user interactions to identify knowledge gaps and updates its knowledge base accordingly.
Benefits:
- Improves accuracy and effectiveness
- Enhances user experience
- Reduces maintenance costs
9. Dialog Management
An AI system must manage the flow of conversation efficiently. This includes deciding when to ask for clarification, when to switch topics, and how to handle complex queries across multiple turns in the conversation.
Example: A user engages with a travel AI assistant to plan a trip. The AI seamlessly transitions between discussing destinations, booking flights, and arranging accommodations.
Benefits:
- Streamlines conversations
- Reduces user frustration
- Enhances user experience
10. Human-AI Collaboration
Many systems require a hybrid model where AI manages routine or simple tasks, while more complex or emotional conversations are handed over to human agents. This collaborative approach ensures efficiency and enhances user satisfaction.
Example: A banking AI chatbot recognizes its limitations in resolving a complex issue and offers to transfer the user to a human customer support agent.
Benefits:
- Ensures resolution of complex issues
- Enhances user satisfaction
- Fosters trust
Conversational AI excellence requires a user-centric approach, combining context awareness, NLU, and personalization. By prioritizing human needs and emotions, developers can create AI interfaces that simplify tasks, provide empathetic support, and drive business success. Continuous innovation and user feedback are key to unlocking conversational AI's full potential.